Manufacturing is a cost-heavy business, so smart manufacturers of all sizes have been looking to find the perfect efficiency formula for their plants. Unfortunately, the results have been mixed, no pun intended.
Many companies historically tried to optimize production processes using established rules of thumb or Excel-like tools for incomplete data analyses. But for most reporting periods, they would find material gaps between realized and expected profits. In our experience, that is due to a lack of more advanced tools and precise measures required to detect and understand the small, real-time variations in the critical variables at each manufacturing step that accumulate and erode returns of a traditional processing facility. And even when the data is available, interpreting and sharing it quickly enough to take effective corrective actions presents a challenge to the shop floor personnel.
Fortunately, new technologies can help manufacturers of all sizes address the underlying issues and (partially) close the profit gaps. These technologies are all part of the 4th Industrial Revolution, known in short as Industry 4.0.
What is Industry 4.0?
The 4th Industrial Revolution is the latest major societal transformation driven by technology and centered on expanding technology into every facet of life. The previous Industrial Revolutions were:
Late 18th to mid-19th centuries: The 1st Industrial Revolution introduced basic division of labor and factory organization. Combined with steam and water power technology, this revolutionized several important industries (and later the world) like textiles;
Late 19th century: The 2nd Industrial Revolution brought rail and communication networks, on top of advances in scientific understanding, which allowed for mass industry with economies of scale;
Mid to Late-20th century: The 3rd Industrial Revolution saw new materials and electronics (namely the transistor) developed. This improved data exchange through telephone networks, computers, and communications satellites.
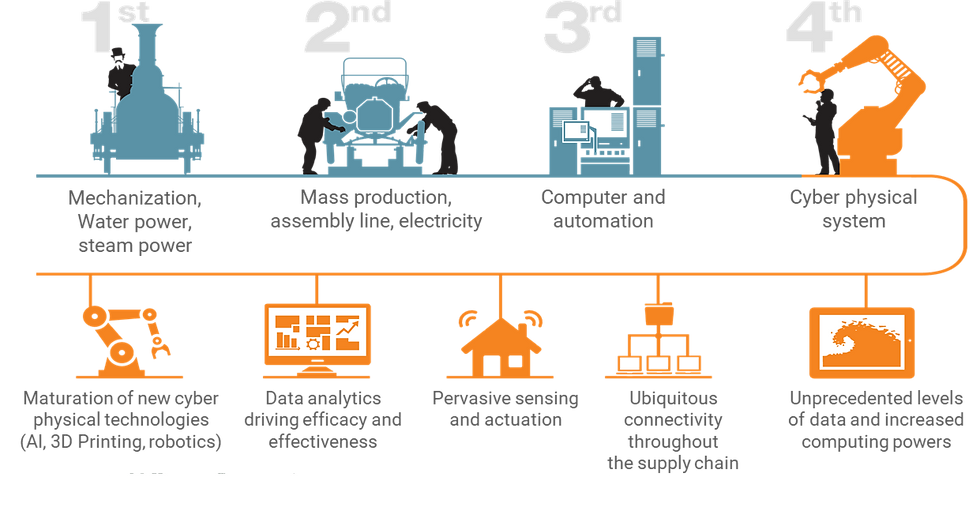
The Fourth Industrial Revolution focuses on developing technologies that extend into every device and facet of life. Lately, manufacturers began to adapt new technologies to the manufacturing environment. The hallmarks of this adaptation are:
Improved data exchange and communications.
Cyber-physical devices and systems.
The Internet of Things or IoT (including wireless connections to machines and sensors).
Cloud computing.
Artificial intelligence (AI).
Often included in or associated with Industry 4.0 are other concepts like smart manufacturing, lights-out manufacturing (or dark manufacturing), the smart factory, and the Industrial Internet of Things (IIoT).
The premise behind leveraging Industry 4.0 technologies in manufacturing is building a network of machines, devices, computers, people, and organizations that can readily and seamlessly communicate and share data in real time. Once built, the network can collect myriads of data points from machine controllers, sensors, RFID badges, hand-held devices, etc., and analyze it all to anticipate issues and changes in production, or to predict the outcomes of changes or adjustments. This leads to better control over the manufacturing process and better decision making.
The ultimate goal of the technology is to develop autonomous systems that can act on their own based on incoming data. This will ultimately improve labor productivity by reducing labor content in manufacturing. Quality and other efficiencies (e.g. material usage and delivery times) will also improve as additional data can predict anticipatory adjustments to systems and processes to keep them optimized. This activity is keyed on using large data sets to identify problems quickly - or even before they occur - to react to them appropriately. Businesses expect large yields from this evolution because these problems have been nearly impossible to solve with traditional methods (or those from the last two or three decades since the high mark of the Third Industrial Revolution).
A complex technology landscape
The landscape surrounding the implementation of Industry 4.0 is complex, with an array of different types of products and services available. As the revolution is in its early stages, there are a lot of new providers, resulting in incredible complexity for businesses choosing a new solution. Some companies offer turnkey solutions, but most produce components or offer systems that address parts of the technology and system. An industry map demonstrates the complexity and how various companies and their products and services overlap:
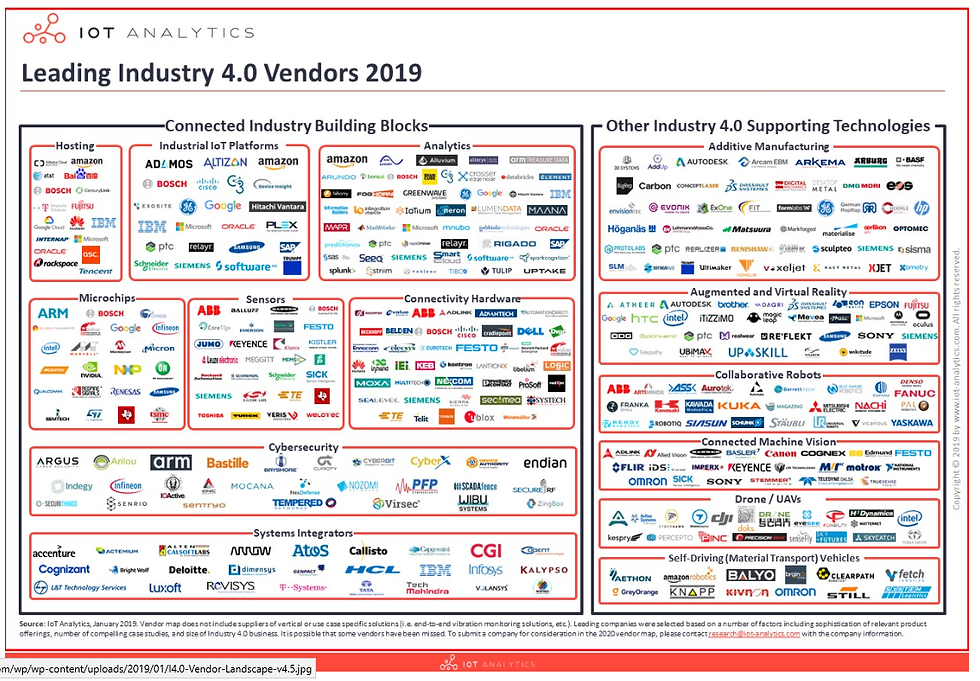
Inevitably, this complexity leads to confusion among customers, resulting in numerous questions about service solutions and vendor caveats.
Yet even a larger issue with realizing the benefits of Industry 4.0 is the costs of implementation, which stems from several components, including:
Investment in new equipment and new systems that contain the needed technology to set up the networks.
The costs associated with interruptions in production as the new technology is placed and integrated into the manufacturing process (in some cases this means new plants which must be built, outfitted, and qualified).
The opportunity cost of lost business while implementation takes place.
Unfortunately for innovative manufacturers, these costs are often significant and beyond the means of many operations below the Fortune 1000 list of companies. This leaves smaller and mid-size manufacturers at a disadvantage with respect to companies that do have the wherewithal to adopt the technology and can continually improve both on a cost and customer service basis. Eventually, these disadvantaged companies will steadily lose business to larger and now more efficient firms or new entrants that are starting from scratch with plant and equipment. Obviously, this leaves these smaller companies in a financially untenable position.
New technologies for old machines
One alternative for the companies is to retrofit existing machinery and systems to build for the type of network, data collection, and analysis that is possible with available Industry 4.0 technology. This is a lower cost solution in terms of both capital outlays and costs associated with interruptions and lost business. However, these retrofits typically do not achieve all of the potential of the technology and still leave companies that choose this path at a disadvantage to companies that fully implement the technology with new infrastructure.
The primary reason for such failures is that most of the manufacturing companies rely on Excel and process data visualization tools to help address process-related issues. While this may work for simpler problems and smaller data sets, especially when there is an existing and known model for problems in the scope, trying to use the same tools and textbook methods in a more complex manufacturing environment is critically hindered by a number of shortcomings:
There is no easy way to set up automatic data pipelines to acquire massive amounts of heterogeneously structured data.
Real-time data is hard to contextualize.
Currently available analytical tools are not suitable for multi-dimensional and/or substantially non-linear problems.
Troubleshooting the data pipeline and (re)running analyses are time-consuming and laborious exercises.
Industrial manufacturing is known to be “DRIP” – Data Rich, Information Poor – so an ability to collect, store, and assimilate large data sets is a critical prerequisite but still is only a means to an end. To harness the power of “big data” and convert it into useful information and operational insights, one needs a suite of different, more advanced analytical methods, notably those falling under an umbrella of artificial intelligence (AI) and machine learning (ML). Such methods are "mapped" to the upper right corner of the Gartner's Analytics Ascendancy Model, depicted below:

IBIS World, a trusted industry research outfit, deems using AI/ML to be “one of the most significant ways in which the manufacturing sector has evolved in the digital era”, based on its recent “Manufacturing in the US” report series.
A three in one solution
What complicates things further is the fact that the most advanced analytical solutions require something beyond good quality low-level machine data and sophisticated algorithms to process it. We are talking about people - data engineers and data scientists - who are instrumental in making these solutions work. Attracting and retaining this hard-to-find and well-paid talent, sought after by many larger “sexier” employers, may present a real challenge for small and mid-market manufacturers.
CPNet provides “solution-as-a-service”, combining all three pillars – data, technology, people – into one offering of a suite of Industry 4.0 technologies, used by a global team of experienced data engineers and scientists to:
Ingest real-time data from machines and extract post-production data from other enterprise systems.
Use robust data contextualization heuristics to perfectly combine streamed machine data and batch-extracted enterprise data.
Run pre-processed data through advanced analytical models powered by machine learning algorithms, designed specifically for manufacturing use cases.
Identify patterns leading to better performance and make them repeatable through delivering timely, human-friendly instructions to the operating teams
The solutions are run on a modern, modular Industrial IoT operating system that is not only customizable for end-to-end configurations but also scalable from 1-2 assets to an entire factory.
Our technology was built entirely in-house and from the ground-up, so all components seamlessly integrate, making it possible to acquire and process data in a cost-efficient manner, both in the cloud and on the edge. Further, the data pipelines’ design enables automated training of the machine learning models, further lowering costs of using the solution over time.

Applications and benefits
AI/ML can bring many benefits to every manufacturer, from local shops to Fortune 500 enterprises. According to IBIS World observations:
"Big data analytics are used by operators to optimize manufacturing processes, increase yield and improve supply chain efficiency. For example, predictive maintenance techniques, which rely on analytics to monitor the condition and performance of equipment during normal operations, are being used by operators to pinpoint and reduce the likelihood of machine failures. Furthermore, AI and machine-learning algorithms are increasingly being used by manufacturers to perform a variety of processes, from demand forecasting and inventory management to yield prediction and defect detection. Through these processes, AI and machine learning programs have enabled manufacturers to improve the end-to-end performance of operations while reducing costs associated with maintenance and transportation and warehousing.”
However, one problem that is faced by any manufacturer running legacy production lines is that these machines are likely not operating at their ideal rate, but just at a rate that was “ideal enough” because of limited resources for experimentation. In absence of major upgrades to production lines and/or changes to input raw materials, engineers would be tasked to figure out the optimal manufacturing recipe about once in two years, to adjust it for the aging asset’s past performance. This is a laborious and expensive task, often prone to human and/or methodological error, so most plants follow a strategy of doing just enough experimentation to identify a good-enough (that is: just profitable) regime of production.
The CPNET platform can effectively run digital equivalents of these experiments as frequently as needed, creating anywhere between 5% and 15% of sustainable gain to gross operating margins, at a very modest cost to the producer. At the intersection, using our software guarantees a more attractive ROI (10x+) and much shorter payback (typically under one year) than buying new capital assets – all at no additional operational risk.
AI doesn’t mean job loss
Last but not least it’s worth noting that adoption of the new technologies, especially those labelled as “AI,” may be compromised by a frequent concern around potential job loss. When employees fear for their jobs, they may be reluctant to embrace a new technology or use it to its fullest extent. Platforms like CPNET, though, are focused on the work that humans can’t do, with the goal not to replace valued specialists but rather augment their manufacturing intelligence. Our platform is built to help manufacturers capture lost profit margin via higher productivity, meaning more opportunity to upskill an existing workforce, hire senior innovators to help the company grow, or reinvest in more machinery, creating more jobs down the line.
This is one of the promises of Industry 4.0 technologies, which are widely seen as the most readily available means to boost revenue per employee, a critical measure of labor productivity that has been essentially stagnant over the past decade.
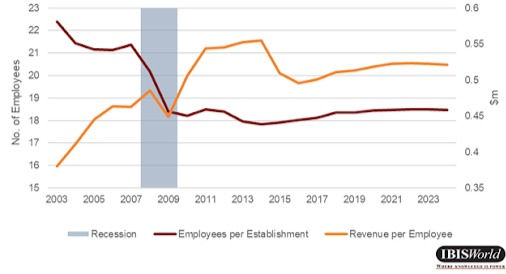
Moreover, advances of these technologies, including big data analytics and AI, are positioned to create over 2 million jobs in the traditional manufacturing industries over the next 5-8 years, according to 2018 estimates from Deloitte and The Manufacturing Institute. Their joint report is prefaced by this bold statement:
“The Fourth Industrial Revolution is transforming the world of work through artificial intelligence, advanced robotics, automation, analytics, and the Internet of Things. Despite common fears, these technologies are likely to create more jobs than they replace—as illustrated by the tight labor conditions in the US and global manufacturing industry.”
Comentarios